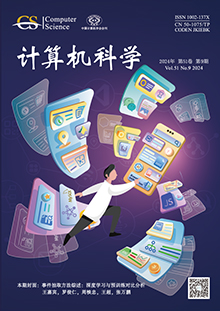
Started in January,1974(Monthly)
Supervised and Sponsored by Chongqing Southwest Information Co., Ltd.
CODEN JKIEBK
Supervised and Sponsored by Chongqing Southwest Information Co., Ltd.
ISSN 1002-137X
CN 50-1075/TPCODEN JKIEBK
Reviewers
Editors
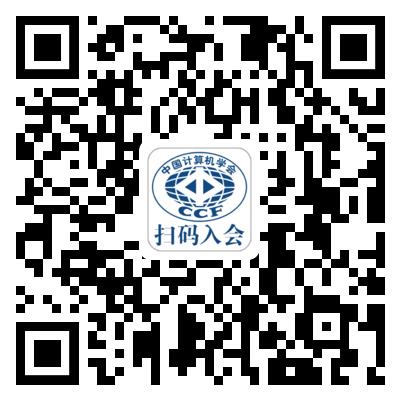
Please wait a minute...
|